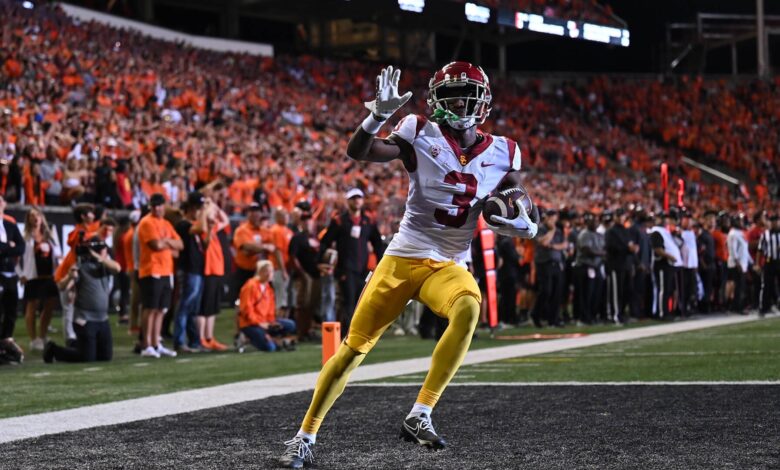
Many dynasty managers look at their teams in three-year windows given the evolving landscape of the NFL. With that in mind, let’s predict the average fantasy points per game for the 2023 class during their first three seasons in the league.
A lot of smart analysts will find one statistic that correlates with future fantasy points and start weeding out players that don’t hit a certain benchmark in that metric. Examples of this would be Relative Athletic Scores (RAS) for tight ends or BMI for running backs. While there is a signal to this, there are complex relationships between multiple statistics that you’d miss by using one filter.
To see if we can determine those relationships, I built an XGBoost model using PFF’s college data. From 2015 to 2022, there are 350 wide receivers whose first NFL season was in this range. This includes both drafted and undrafted players. The benefit of using a machine learning model is that they are uniquely suited to finding complex patterns between variables. The downside is that 350 is a very small number of players for a model to learn these patterns on. So, like with any model, we’ll need to provide additional context to its results.
The model uses 18 variables to try to predict future wide receiver fantasy points. They can be split into 3 groups: best season production, play style, and general. Best season production includes a player’s top mark across their college career in things like targets per route run, yards per game, and touchdown rate, among others. Playstyle includes things like how often they line up in the slot and their average depth of target. The general group includes where they were selected in the draft, their college conference, and the number of college games played. A wide receiver’s draft pick is particularly important as we’ll see below. I’m currently using NFL Mock Draft Database’s consensus big board for the 2023 class.

You’ll notice I don’t have any Combine metrics included. The benefit of excluding them is that we can keep all players that weren’t invited to the combine or didn’t take part in some or all drills in the dataset. The downside is that these variables aren’t helping the model make predictions. Yet, Kevin Cole of Unexpected Points has great data that shows Combine statistics for WRs are much more predictive of their draft selection (something we have already included) than their actual NFL value.
So what variables matter more for future NFL fantasy points? Below are two bar charts that show the model’s gain or relative contribution to the model by variable. A higher number indicates that a variable is more important for prediction. Each position has a very different split, with a player’s selection in the NFL draft being relatively more important for WRs than any other offensive skill position.
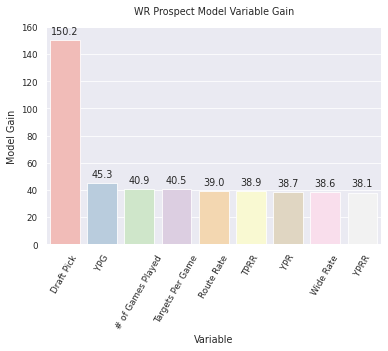
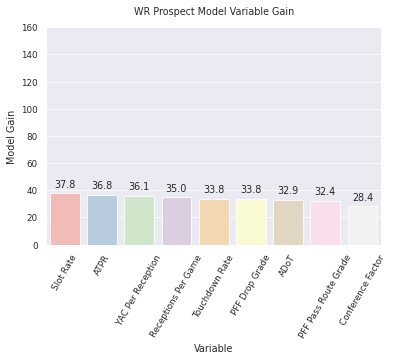
In general, draft capital is the most important variable, with simple statistics like yards per game, targets per game, and the number of college games played (a proxy for both early declare status and injuries) leading the way from there. Yet, as you can see, most variables beyond draft capital have a relatively similar gain amount. So, selecting just one of them to weed players out on doesn’t seem like the best process.
Now let’s see how the model actually performs. I held last year’s class out of the model-building process to get a sense of how good it is at predicting future fantasy points.
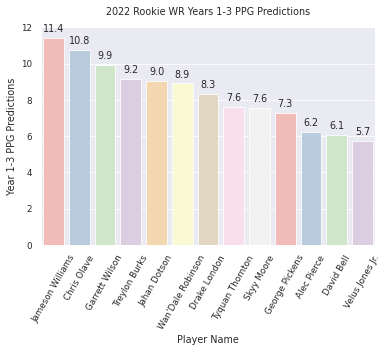
The 2022 class has only had 1 NFL season, but we already have an updated sense of how they’ll perform. The jury is still out on Jameson Williams given how injured he was in 2022. We had that additional context going into rookie drafts that the model did not have. Being higher on Chris Olave than the market looks like a great call so far, along with also having Garrett Wilson ranked highly. Even if the Drake London hate went a bit too far, I’ll take having Olave and Wilson as the top two wide receivers (given Jameson’s injury) going into rookie drafts last year. Now let’s take a look at 2023.
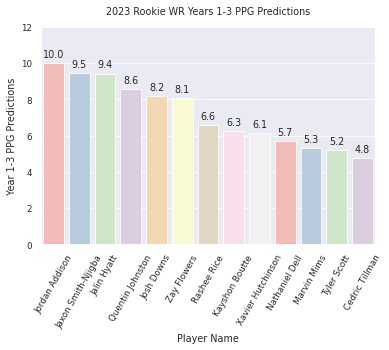
The biggest surprise here is probably Addison over JSN. Again, the model doesn’t have the Combine context of Addison’s relatively slow 40-time given his weight or JSN destroying the agility drills. This might impact their ultimate draft selection, which would adjust their predicted fantasy points. Similarly, if injuries prevent sustained production for a player, this also has to be considered. As an example, Brian Ford provided some great context surrounding Cedric Tillman. In general, though, the 2023 predictions look reasonable. And we were able to do it in a way that incorporates a lot of different production variables without weeding out players on one specific stat. I’ll be back next to show what variables actually matter for future running-back fantasy points.
ATTN Dynasty Commissioners: Do you want to do something cool for your league? How about a 1-hour live show dedicated to YOUR league? Team-by-team breakdowns, rankings, and more. For details and to book a show, visit: GoingFor2.com/plp.